What Is Streaming Data? Frequently Asked Questions About Data Streaming
Streaming data is a rapidly evolving field. In this article, we answer the most frequently asked questions about why, how and when to use data streaming technology.
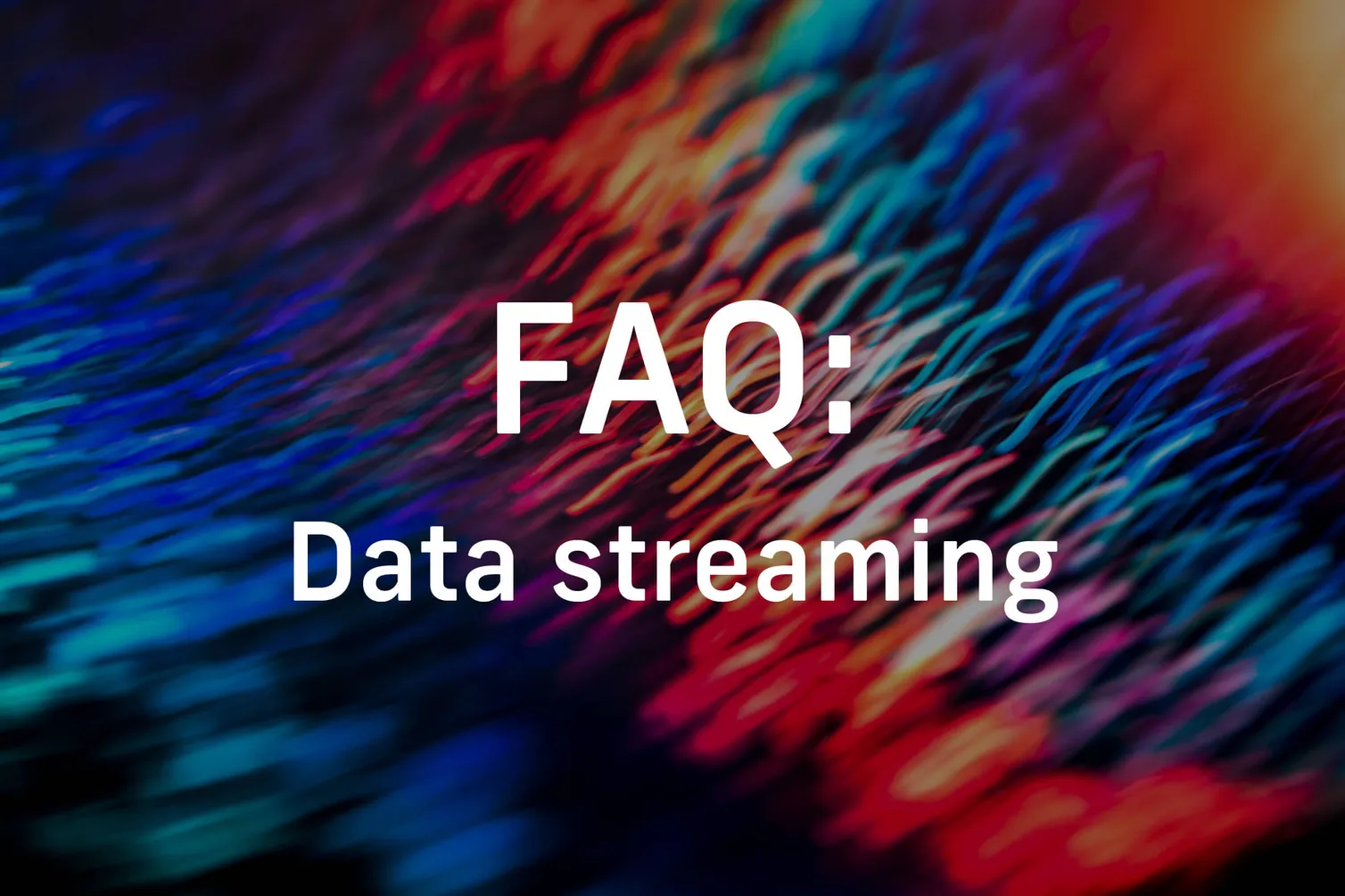
Data streaming is a complex topic, partly because there are so many ways to describe it. You’ve probably seen references to “streaming data,” “data analytics,” “real-time data,” “data pipelines,” “message brokers,” “pub/sub messaging,” and similar terminology.
Many of the terms we’ll discuss are used interchangeably or flexibly because the technology is constantly evolving, so don’t worry about memorizing definitions. Instead, focus on understanding the core process and technology concepts that enable organizations to harness the power of streaming data in real-world applications.
This article covers the most frequently asked questions about streaming data.
What is a data stream?
As the name implies, a data stream consists of data that continuously flows from a source to a destination to be processed and analyzed in near real time. Think about the continuous arrival of data relating to stock prices or the task of finding the closest car for transportation.
While the noun “data stream” describes the character of data itself, the verb “data streaming” refers to the act of producing, or working with, such data. Again, you might see these used interchangeably, even if that doesn’t make linguistic sense. Regardless, we should all know what we’re talking about when referring to “data streams”, “data streaming” or “streaming data.”
How does data streaming work?
A complete answer to this question involves a detailed description of several complex technologies. But at a high level, data streaming usually involves the following:
- One or more data sources produce continuous streams of data.
- A message broker, such as Apache Kafka, groups these streams into topics, ensuring consistent ordering. Kafka also uses replication and can distribute data across multiple servers to provide fault tolerance.
- Applications consume data from these topics, process it, and act on it accordingly.
- The results of processed data can be streamed back to the message broker for further distribution.

The benefits of streaming data
In our article on stream processing trends, we cover the benefits of streaming in more detail, but here’s a recap of those benefits:
- Enable better business decisions: Data stream processing enables digital leaders to make decisions based on what’s happening now. These insights impact decisions across the organization: customer support, fraud detection, supply chain management, resource scheduling, machine maintenance, marketing and pricing, product development and more.
- Increase the value of AI, ML and automation investments: Streaming data makes AI, ML, automation, and anything else that relies on data more responsive by removing the inherent latency in working with batch uploads and data lakes.
- Deliver data-driven personalized user experiences: Using streaming data enables marketing, customer support and product development to align with and act on customer behavior in real time, improving the customer experience and opening up opportunities for sophisticated data-driven apps.
- Cut data storage costs: Stream processing technologies enable companies to process data “in-memory,” reducing reliance on data storage and reducing compute costs.
Overall, the benefits of streaming data provide businesses with a competitive edge by enabling them to harness the power of data in real-time for improved decision-making, operational efficiency, and customer experience.
Why is streaming data so hard to handle?
Given the benefits of streaming data, you’d think more companies would do it. Unfortunately, there are a lot of hurdles you need to overcome before you can reap these benefits. Our Chief Technology Officer explains this is detail, in his post “why streaming data technologies are so hard to handle “ but here’s a quick summary.
The main reasons why streaming data is difficult to handle are the complexity of microservices integrations, the steep learning curve for most software engineers, and the specialization required for handling real-time data (such as windowing, parallel processing, handling out-of-order events and so on).
Most streaming technologies available today, such as Apache Kafka and Pulsar, are challenging to use, especially for smaller organizations with fewer in-house Java or Scala developers (or none at all). This is because they require a high level of expertise in streaming data infrastructure, which most software engineers do not have. Furthermore, integrating ML into streaming data is even more complicated, as it requires a broad spectrum of skills across various software disciplines.
Another challenge is handling big data at scale, which requires a completely different architecture than traditional databases. Developers need to filter through vast volumes of data to find relevant information, and ensure that data remains in context within the stream.
However, there’s hope on the horizon. While streaming data is undeniably challenging, platforms like Quix are working to simplify the process and make it more accessible to developers and organizations, ultimately helping to drive innovation and transformation across various industries.
How does data streaming compare to batch processing?
Traditional batch processing involves working with a fixed set of data, collected over time, which is analyzed together at a certain point. It does not seek to take action — or even analyze data — in real time. Batch processing might be suitable when working in real time doesn’t matter, when dealing with huge volumes of data, or when analysis requires a large amount of data simultaneously.
Batch processing typically occurs at a convenient interval, such as daily or weekly. This may be to align with other external dependencies, such as the close of business in retail or the close of the stock market in financial services and banking. It might also be scheduled at a convenient time when resources are more available, such as during overnight downtime.
Meanwhile, data streaming is a process that never sleeps. Companies that require real-time data to make decisions and those that want to process data as soon as it is created will benefit from streaming data analytics.

What are data streaming applications?
Streaming data applications are those designed to process a continuous incoming data flow. Such applications may process real-time data, act on streaming data, or produce new data from the incoming data. Data streaming applications often work at a large scale, but that’s not a requirement. The most significant aspect of streaming applications is that they act in real time.
Keep in mind that it’s easy for an application to be decoupled from its data source so that streaming data may be processed by a separate entity than the one producing the data. A data streaming application — or components of it — may also be able to work with batched data. This can be of great use when developing or testing an application.
What is a streaming data pipeline?
A pipeline enables data to flow from one point to another. It’s software that manages the process of transporting that data. A pipeline should help prevent common problems such as:
- data corruption
- bottlenecks
- loss of data
- duplicated data
A streaming data pipeline does this job in real time, often with extensive data. Compared to a data streaming application, which can have various processing tasks, a pipeline’s task is straightforward: move data from point A to point B.
What is streaming data analytics?
Also known simply as streaming analytics or event stream processing, this analyzes real-time data via event streams using continuous queries.
This processing can involve:
- aggregation, such as the summing or averaging of values
- transformation of values from one format to another
- analysis, in which future events are planned or predicted
- enrichment of data by combining it with data from other sources to add context
- ingestion, by sending the data to a permanent store such as a database
How does machine learning work with streaming data?
Machine learning (ML) is an artificial intelligence that uses data for self-improvement. ML models are trained on existing data sets to process further data according to what they learn.
Traditionally, ML models are trained as part of an offline process, a type of batch processing. But data streaming technologies provide an opportunity to improve existing processes. With data streaming, ML models can be trained continuously, analyzing each piece of data as it is created. The models’ algorithms then improve in real time, with far more accurate and relevant results.
Related reading: Learn how to deploy your own traffic monitoring app using computer vision and ML
Can I work with streaming data using Python? Is it better than C#?
Yes, and maybe. Ultimately, the language choice is yours to make, but both are entirely possible. Python is popular with data scientists. It has excellent support for data handling via libraries such as Pandas, Bokeh, and NumPy.
C# is popular within the Microsoft ecosystem. The Quix Streams library has bindings for both Python and C#. Quix Streams abstracts out a lot of complexity, so it‘s easy to use either language. We also offer APIs, which any HTTP-capable language can use.
If I stream my data, will I lose it once I’ve analyzed it?
No, just because you’re streaming data in real time doesn’t mean you have to use it or throw it away immediately. Some message brokers like RabbitMQ deliver a message, then it’s gone for good. But Kafka supports permanent data storage. You can also build your architecture to store data later in the pipeline.
The Quix platform allows you to persist data on a topic-by-topic basis (our head of Platform explains how to persist data efficiently in this post). This provides a compromise, allowing you to store your most important data permanently without wasting resources.
Do I need to use Kafka for data streaming?
Apache’s Kafka framework dominates the data streaming landscape. You’ll see it often mentioned as the industry leader. While nothing requires you to use Kafka, you should at least understand its purpose and capabilities and evaluate it as a candidate for a data streaming architecture.
The Quix platform uses Kafka, although it doesn’t depend on it. We simplify working with Kafka, a highly complex product that can require a lot of engineering resources to set up and manage. Quix manages Kafka for you as part of our platform, so you don’t have to invest heavily in infrastructure.
What are some tools for working with data streams?
There are many powerful tools available that can help with handling data streams efficiently and effectively. We’d need a whole separate article to help you choose one, because the choice depends on the complexity of your use case. Nevertheless, here is a list of some popular tools to help you get started:
Apache Kafka
Apache Kafka is a distributed streaming platform that is widely used for building real-time data pipelines and streaming applications. It is designed to handle high-throughput, fault-tolerant, and scalable data streams, making it ideal for processing large volumes of data in real-time. Kafka provides durable message storage, event-driven architecture, and seamless integration with its complimentary stream processing tools such as Kafka Streams and ksqlDB.
Apache Flink
Apache Flink is a stream processing framework that provides event time processing, fault tolerance, and state management capabilities. It supports processing data streams in real-time and batch mode, making it a versatile tool for handling data streams of any size. Flink also offers a wide range of connectors for data sources and sinks, including Kafka, Apache Cassandra, Amazon S3, and more.
Amazon Kinesis
Amazon Kinesis is a fully managed service by Amazon Web Services (AWS) for collecting, processing, and analyzing streaming data in real-time. It provides capabilities for ingesting data from various sources, such as IoT devices, social media feeds, and clickstream data, and allows you to process and analyze the data using AWS analytics services like Amazon Kinesis Data Analytics and Amazon Kinesis Data Firehose.
Redpanda
Redpanda is a modern, high-performance, Kafka-compatible event streaming platform designed to handle real-time data processing and analytics use cases. It aims to provide a simple, reliable, and efficient solution for building distributed systems that require high-throughput and low-latency data streaming capabilities. Redpanda’s Wasm engine offers a flexible and powerful way to implement custom stream processing logic using familiar programming languages and run them directly within the Redpanda cluster.
Learn how Redpanda compares to Kafka
Google Cloud Dataflow
Dataflow is a fully managed service for stream and batch data processing that allows you to build, deploy, and manage data processing pipelines. It is based on the Apache Beam programming model, providing a unified API for both batch and stream processing. Dataflow can read and write data from various sources and sinks, including Google Cloud Pub/Sub.
Quix
Quix is a fully managed stream processing platform coupled with an open-source stream processing library. Quix specializes in simplifying data processing for data-intensive applications. It offers a developer environment for building, testing, and deploying streaming applications, enabling users to quickly develop data pipelines and derive insights from real-time data streams using Python or C#.
Should my company use streaming data?
Ultimately, only you can answer that strategic question, and migrating from batch processing to stream processing can require a paradigm shift for your whole organization.
You’ll be able to best compare batch data processing vs. streaming data processing by considering the following:
- How soon does the data you work with become stale? Is there value in analyzing this data as quickly as possible? Real-time data streaming is as fast as it gets. You can also consider that your data doesn’t have to arrive strictly in real time to benefit from the streaming model. Some elements of data streaming can be used to improve your architecture and reduce processing and storage costs — even if you only process data in micro-batches.
- Can you meaningfully analyze individual data points in isolation? If not, data streaming might prove to be complicated.
- How much engineering time do you have to spare to set up a data streaming architecture? (Hint: Quix can help!)
- Are you working with massive data sets that cannot be processed in real time? It’s unlikely, but it’s possible.
What’s the easiest practical way to understand data streaming?
If you’ve read this FAQ from beginning to end, you might feel overwhelmed. There are many concepts to absorb, and the theory of data streaming is only one part of the puzzle.
Hopefully, you’re interested in the practicalities of data streaming too.
We’ve engineered the Quix platform to make data streaming accessible — even without a massive team of infrastructure engineers — so whether you’re an experienced data scientist, a digital product developer, or a machine learning student, you can get started quickly. Please reach out to our friendly engineers on Slack if you have questions. We love hearing from you.
What’s a Rich Text element?
The rich text element allows you to create and format headings, paragraphs, blockquotes, images, and video all in one place instead of having to add and format them individually. Just double-click and easily create content.
Static and dynamic content editing
A rich text element can be used with static or dynamic content. For static content, just drop it into any page and begin editing. For dynamic content, add a rich text field to any collection and then connect a rich text element to that field in the settings panel. Voila!
How to customize formatting for each rich text
Headings, paragraphs, blockquotes, figures, images, and figure captions can all be styled after a class is added to the rich text element using the "When inside of" nested selector system.
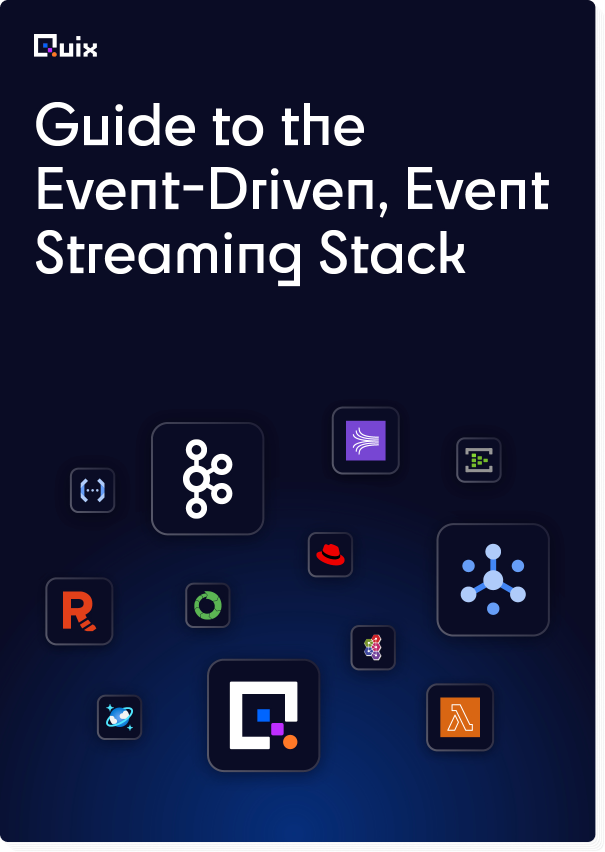
Check out the repo
Our Python client library is open source, and brings DataFrames and the Python ecosystem to stream processing.

Interested in Quix Cloud?
Take a look around and explore the features of our platform.

Interested in Quix Cloud?
Take a look around and explore the features of our platform.
