Optimizing manufacturing efficiency with streaming data and ML
How CloudNC makes better predictions for maintenance, generates insights with very low latency, and transforms its factory operations with streaming data.
.jpg)
4x
75%
5%
Quix has given us an environment to handle a huge amount of real-time machine data.

When you need a part, you go to the hardware store. But when industries that build anything from smartphones to spaceships need highly technical, custom parts, they go to CloudNC to manufacture to their specifications.
The London-based company, named by the World Economic Forum as one of the 100 most promising technology pioneers of 2021, is at the forefront of a manufacturing revolution that uses high-frequency time-series data to optimize the manufacturing of precision parts. Its mission is to revolutionise manufacturing by making it 10x more efficient, sustainable and faster.
The opportunity
Optimize manufacturing efficiency
CloudNC’s ambition to deliver autonomous manufacturing to the world relies on an optimized workflow that can take in customer specifications for a metal part, autonomously calculate how to machine it, and then optimally schedule its fabrication and ship it as quickly as possible.
“We’re trying to remove as many hours as possible between customer design and delivery,” says Chris Angell, Lead Systems Engineer for CloudNC. Inside the factory, their goal is to maximize the number of jobs a CNC machine can produce, while minimizing the hands-on process and time wasted when a machine is idle or breaks down.
These are the goals that CloudNC identified, to make the process more efficient and the challenges they are currently facing that prevent their achievement:
- Continually update factory schedules based on current machine performance
One way to improve the manufacturing process is fabrication scheduling which requires real-time data. Factory software used by CloudNC gives high-level stats, but the data is delayed and missing vital information. CloudNC wants to control its data, but its existing batch-processing software prevents it from owning or easily accessing detailed data. - Predictive maintenance to prevent breakdowns
Everything needs maintenance. But when the parts made and materials used keep changing, the stress on the specialized machines varies greatly, making it nearly impossible to predict when they need servicing.
By gathering real-time data, CloudNC can track the use of each tool to predict the optimal time to change it. - Real-time reaction to early warning signs
A strong indicator of something going wrong in a CNC machine is vibration. It can suggest the machine is about to break down, or perhaps a defective piece of metal is being machined. In any case, it’s time to press pause and take corrective action.
It’s important to get information from the machines on the factory floor to the agent with minimal latency. It doesn’t help to batch process information about vibrations from yesterday or even a few minutes before. This needs immediate alerting to run more efficiently with less downtime. - Optimizing how parts are created through machine learning
When a customer asks CloudNC to make a part, they provide the 3D model and then CloudNC’s team uses an ML model to calculate the optimal cutting paths to produce this part. CloudNC wants to start to gather data on the manufacturing process and fine-tune the model’s instructions and parameters. At the moment, they don’t use this information at all
The solution
Using streaming data and ML to provide real-time monitoring and alerting
All the goals above require that CloudNC transition from an old-factory model of batch-based utilization analytics to modern stream processing systems that utilize machine learning to unlock value in their data.
After evaluating different vendors, CloudNC decided to go for Quix: Quix enables CloudNC to ingest and process very high-frequency time-series data in order to perform real-time monitoring and alerting, with the option to store data as needed.
CloudNC’s time-series streaming data architecture
It starts with a Linux box on the factory floor, which uses the Quix Streams client library to ingest high-frequency time-series data from each machine to the cloud in a very efficient format.
Real-time value is generated from the data using stream processing with results stored and consumed immediately by downstream systems. Data is kept in memory throughout the entire pipeline, ensuring the solution is efficient and low latency.
All raw and processed time-series data is stored in Quix where it creates long-term value from batch analytics, which is executed at regular intervals against historic data in the platform, as well as for developing, training and testing machine learning models.CloudNC started using Quix on simple parts analytics, such as recording machine cycle times by detecting patterns in the time-series data. This has grown to include Quix at the heart of both streaming and batch data analytics, enabling it to generate value from Quix using the Query API while moving toward higher-value real-time applications.
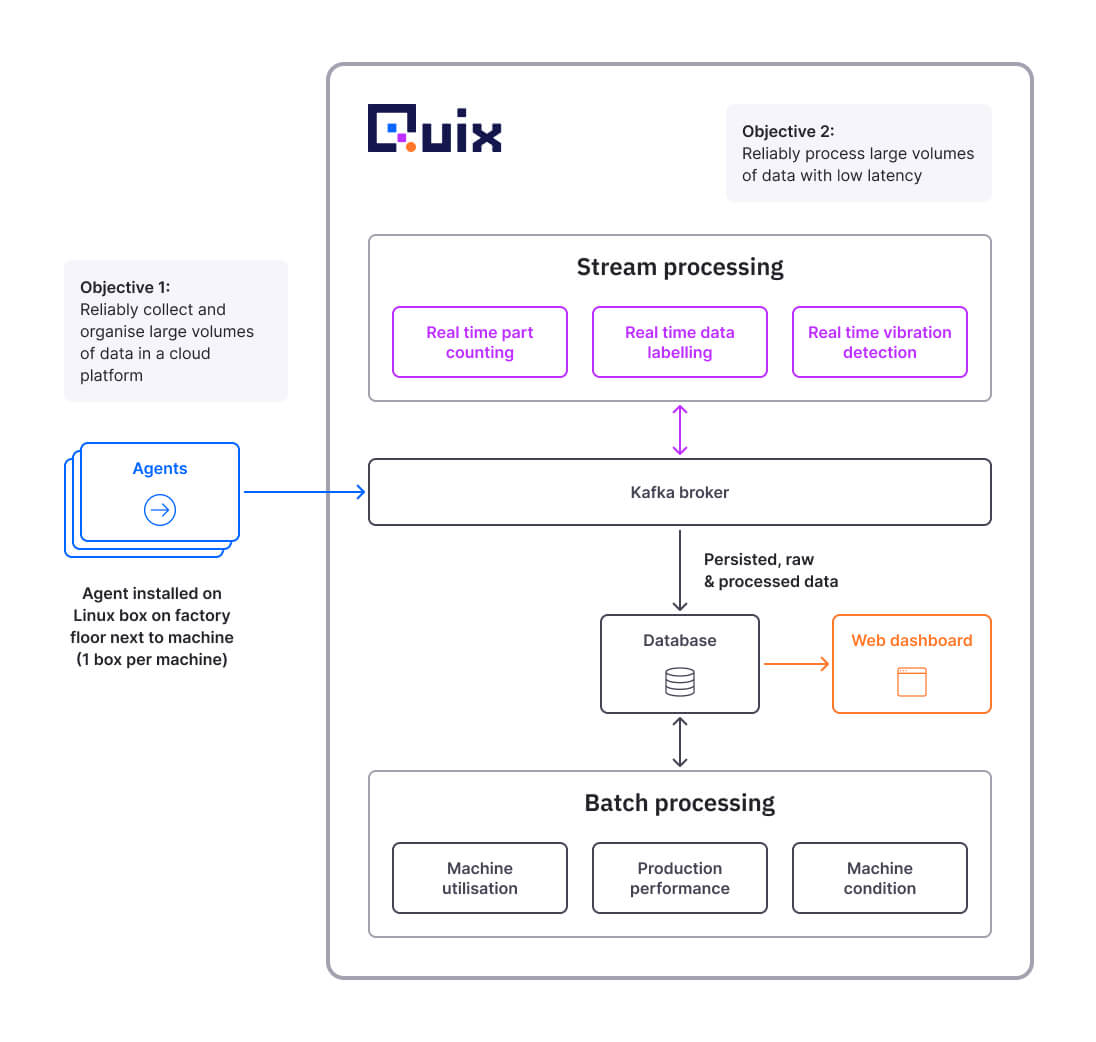
The outcome
The entire solution was developed by two CloudNC engineers in just 4 weeks
By leveraging Quix, theCloudNC team was able to build real-time event streaming applications on their own time-series data 4 times faster because no lengthy infrastructure projects were required. This saved 16 weeks of development effort, precious resources which were reassigned to other important projects.
Ultimately, CloudNC estimate a 5% efficiency gain from implementing stream processing using Quix by reducing manual operations and reducing machine downtime.
CloudNC uses Quix to:
- Optimize production efficiency
By constantly monitoring the tools, their fabrication capacity, and the count of parts that have been delivered so far, CloudNC can make better use of its machines to run at peak capacity and therefore offer more reliable scheduling with lower overheads. - Make better predictions for maintenance
CloudNC uses Quix to understand wear and tear. That includes monitoring things like the forces applied, material density and other conditions to generate a physics-based predictive model for wear and tear. As a result, CloudNC can plan for a reasonable lifespan for a drill bit and replace it just before it’s worn out, rather than waiting until it breaks — and suffering repair time delays. - Detect small problems before they cause big trouble
Quix enables CloudNC to handle very high-frequency time-series vibration data in order to perform this real-time monitoring and alerting - Optimize how parts are created through machine learning
Quix enables CloudNC to gather data on the manufacturing process and fine-tune the model’s instructions and parameters. Now Quix has given us the environment to finally manage that information, to look at it, store it, or act on it immediately.
Their ultimate goal is to use autonomous technology to optimize the machine learning model by automatically retraining it with more current data.
It started with solving a simple problem — that people tended to be unreliable about recording when parts were produced — and has expanded to using high-frequency time-series data across the organization. The breadth of data available and ease of access are opening up new opportunities for the team.
What’s a Rich Text element?
The rich text element allows you to create and format headings, paragraphs, blockquotes, images, and video all in one place instead of having to add and format them individually. Just double-click and easily create content.
Static and dynamic content editing
A rich text element can be used with static or dynamic content. For static content, just drop it into any page and begin editing. For dynamic content, add a rich text field to any collection and then connect a rich text element to that field in the settings panel. Voila!
How to customize formatting for each rich text
Headings, paragraphs, blockquotes, figures, images, and figure captions can all be styled after a class is added to the rich text element using the "When inside of" nested selector system.
Quix has given us an environment to handle a huge amount of real-time machine data.

Ready to get started?
Learn more about how companies are building data integration pipelines with Quix.
Related content
